ZenML offers the capability to build end-to-end ML workflows that seamlessly integrate with various components of the ML stack, such as different providers, data stores, and orchestrators. This enables teams to accelerate their time to market by bridging the gap between data scientists and engineers, while ensuring consistent implementation regardless of the underlying technology.

Harold Giménez
SVP R&D at HashiCorp
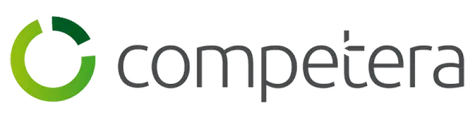
ZenML's automatic logging and containerization have transformed our MLOps pipeline. We've drastically reduced environment inconsistencies and can now reproduce any experiment with just a few clicks. It's like having a DevOps engineer built into our ML framework.
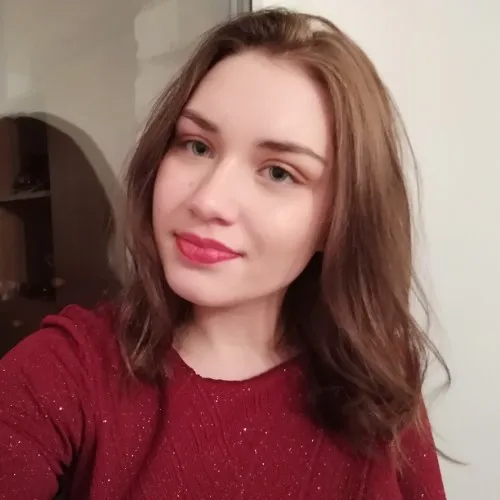
Liza Bykhanova
Data Scientist at Competera
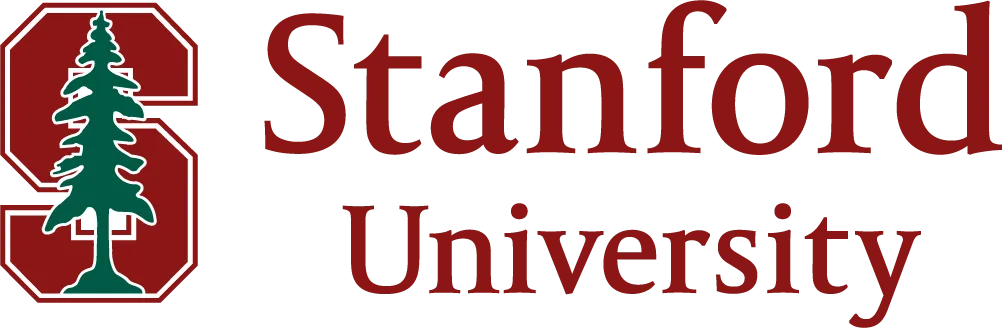
"Many, many teams still struggle with managing models, datasets, code, and monitoring as they deploy ML models into production. ZenML provides a solid toolkit for making that easy in the Python ML world"

Chris Manning
Professor of Linguistics and CS at Stanford
"ZenML has transformed how we manage our GPU resources. The automatic deployment and shutdown of GPU instances have significantly reduced our cloud costs. We're no longer paying for idle GPUs, and our team can focus on model development instead of infrastructure management. It's made our ML operations much more efficient and cost-effective."
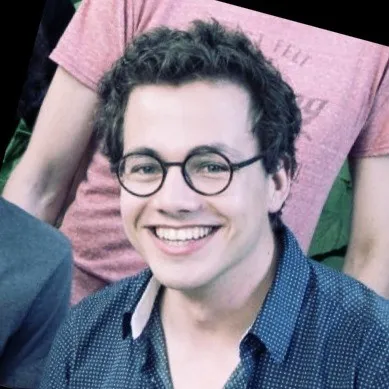
Christian Versloot
Data Technologist at Infoplaza
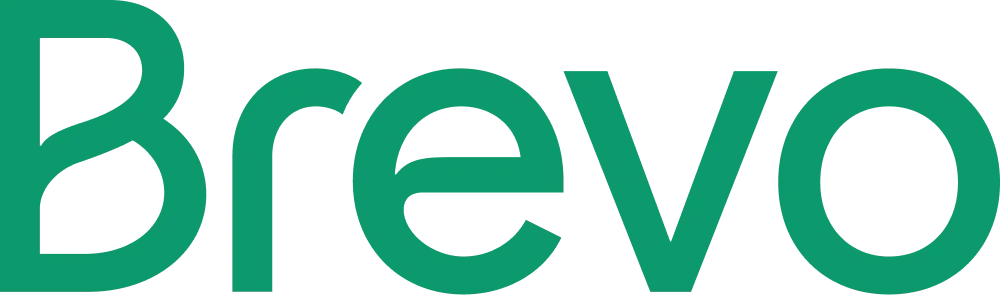
"After a benchmark on several solutions, we choose ZenML for its stack flexibility and its incremental process. We started from small local pipelines and gradually created more complex production ones. It was very easy to adopt."
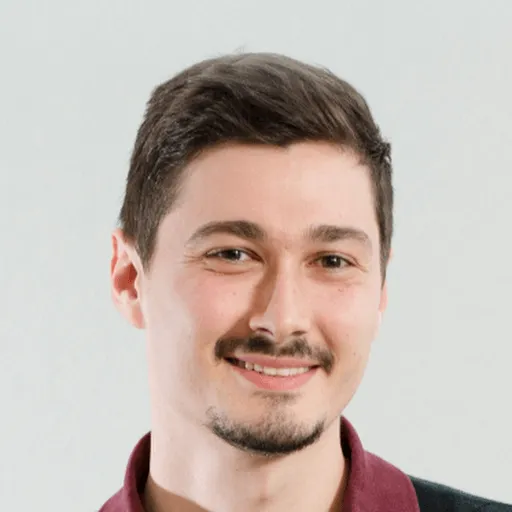
Clément Depraz
Data Scientist at Brevo
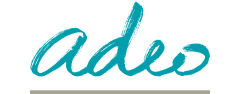
"ZenML allowed us a fast transition between dev to prod. It’s no longer the big fish eating the small fish – it’s the fast fish eating the slow fish."
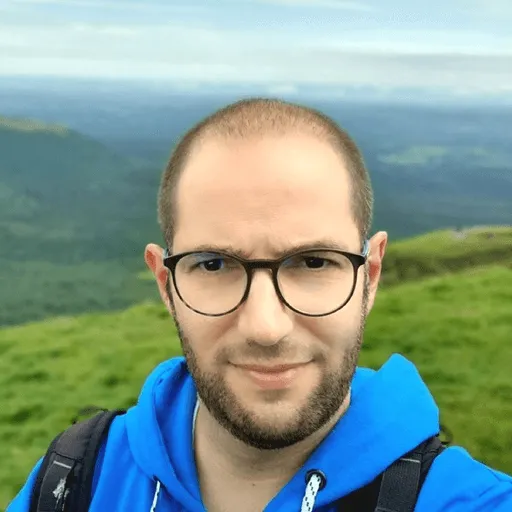
François Serra
ML Engineer / ML Ops / ML Solution architect at ADEO Services

"ZenML allows you to quickly and responsibly go from POC to production ML systems while enabling reproducibility, flexibitiliy, and above all, sanity."

Goku Mohandas
Founder of MadeWithML
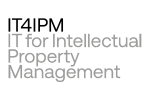
"ZenML's approach to standardization and reusability has been a game-changer for our ML teams. We've significantly reduced development time with shared components, and our cross-team collaboration has never been smoother. The centralized asset management gives us the visibility and control we needed to scale our ML operations confidently."
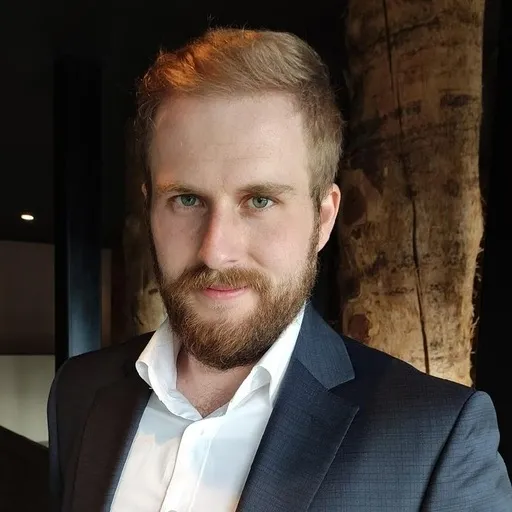
Maximillian Baluff
Lead AI Engineer at IT4IPM
"With ZenML, we're no longer tied to a single cloud provider. The flexibility to switch backends between AWS and GCP has been a game-changer for our team."
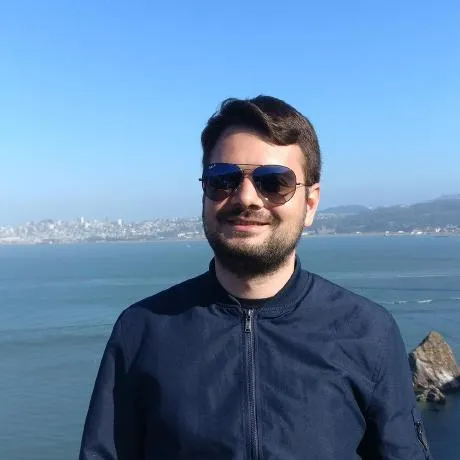
Dragos Ciupureanu
VP of Engineering at Koble
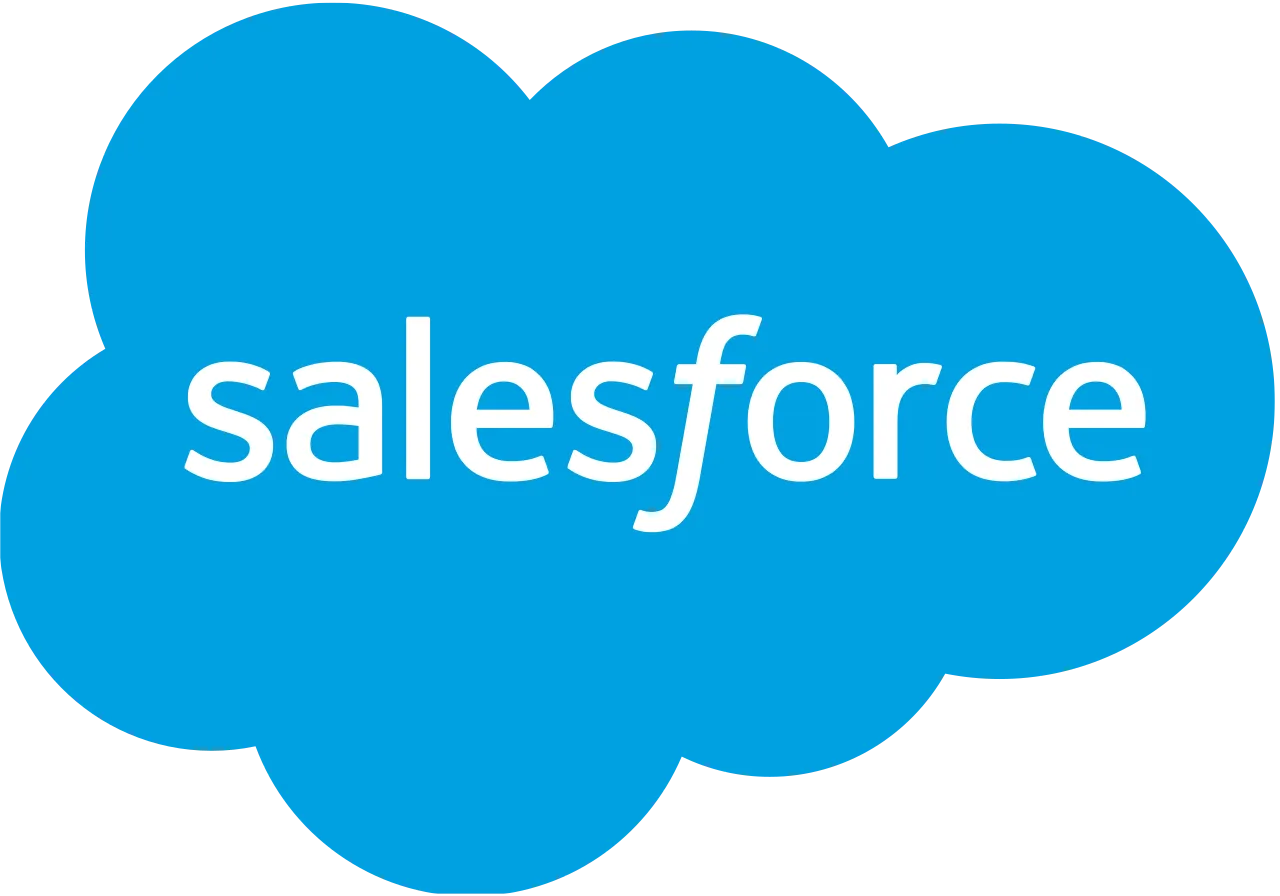
"ZenML allows orchestrating ML pipelines independent of any infrastructure or tooling choices. ML teams can free their minds of tooling FOMO from the fast-moving MLOps space, with the simple and extensible ZenML interface. No more vendor lock-in, or massive switching costs!"

Richard Socher
Former Chief Scientist Salesforce and Founder of You.com
Thanks to ZenML we've set up a pipeline where before we had only jupyter notebooks. It helped us tremendously with data and model versioning and we really look forward to any future improvements!

Francesco Pudda
Machine Learning Engineer at WiseTech Global